The Power of Agriculture Dataset for Machine Learning
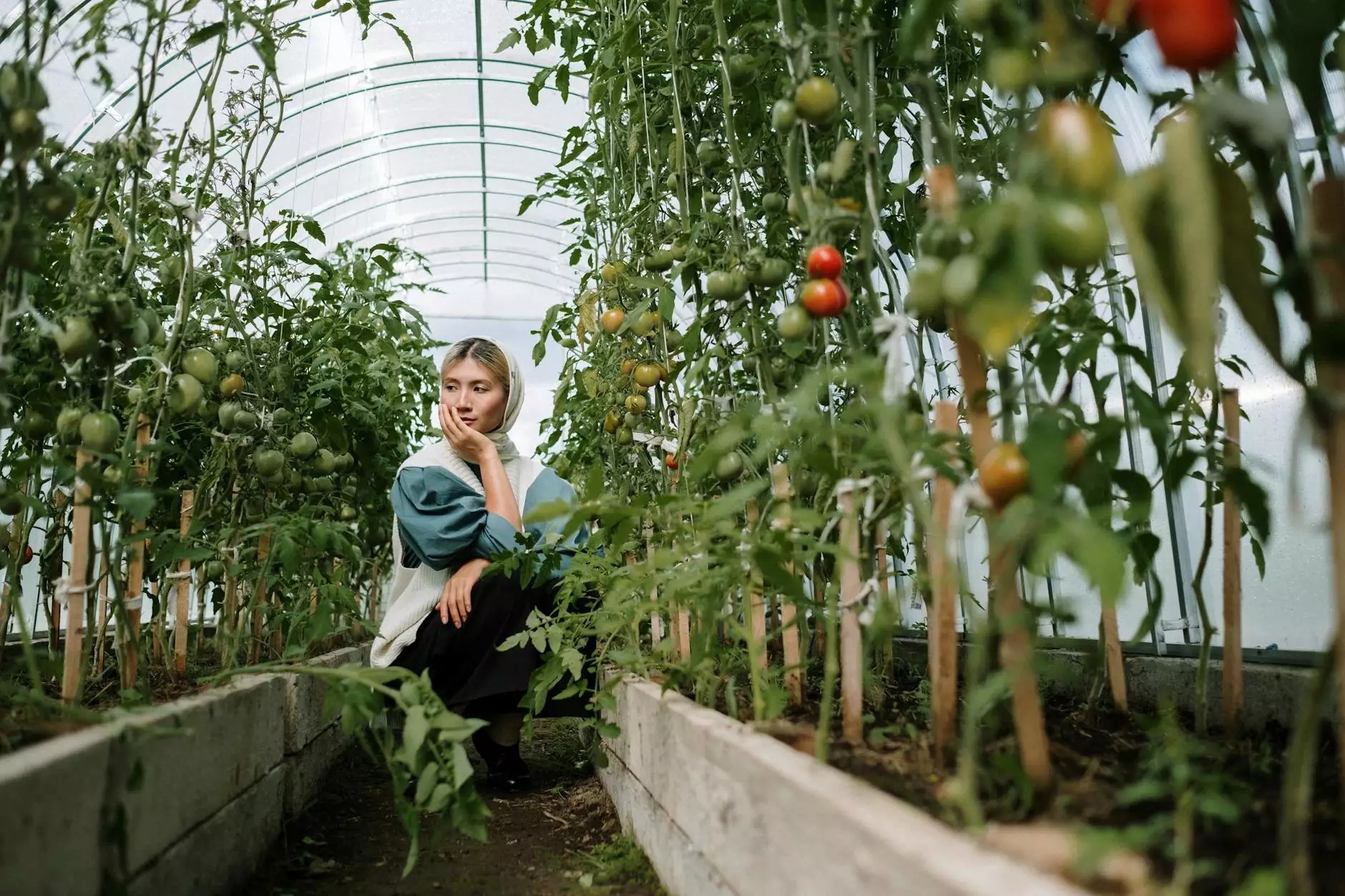
In today's rapidly evolving world, technology plays a crucial role in transforming various industries and sectors. Within the realm of agriculture, the integration of machine learning techniques has paved the way for groundbreaking advancements that are revolutionizing modern farming practices.
Unlocking Insights with Agriculture Dataset for Machine Learning
One of the key pillars of leveraging the potential of machine learning in agriculture is the availability of high-quality datasets. These datasets encompass a wide range of information related to crop yields, soil quality, weather patterns, pest infestations, and more. By analyzing and processing this data using advanced machine learning algorithms, farmers and agricultural practitioners can gain valuable insights that drive informed decision-making.
Enhancing Crop Yield Predictions
By harnessing the power of agriculture dataset for machine learning, farmers can optimize their crop management strategies to enhance yield predictions. Machine learning models can analyze historical data on crop performance, environmental factors, and agricultural practices to forecast future yields with remarkable accuracy. This predictive capability enables farmers to preemptively address challenges and maximize productivity.
Improving Soil Health Monitoring
Another critical application of machine learning in agriculture revolves around soil health monitoring. Through the utilization of sophisticated sensors and data collection tools, farmers can gather extensive datasets on soil composition, moisture levels, nutrient content, and microbial activity. Machine learning algorithms can then process this data in real-time to offer precise recommendations for soil management practices, leading to improved crop health and sustainability.
Optimizing Resource Allocation
The implementation of machine learning in agriculture extends beyond yield predictions and soil health monitoring to optimize resource allocation. By analyzing datasets on water usage, fertilizer application, and power consumption, farmers can identify areas where resource efficiency can be enhanced. This data-driven approach enables the judicious allocation of resources, resulting in cost savings and environmental sustainability.
Ensuring Pest and Disease Management
One of the most significant challenges in agriculture is combating pests and diseases that threaten crop health and productivity. Advanced machine learning models trained on extensive agriculture datasets can identify patterns and anomalies associated with pest infestations and diseases. By detecting potential outbreaks early and recommending targeted intervention strategies, farmers can mitigate risks and protect their crops effectively.
Conclusion
In conclusion, the integration of machine learning techniques fueled by comprehensive agriculture datasets has the potential to reshape the future of farming. By harnessing the power of data analytics and predictive modeling, farmers can make informed decisions, optimize resources, and enhance sustainability. Leveraging the insights derived from agriculture datasets for machine learning will not only drive efficiency but also foster innovation and resilience in the agricultural sector.