Understanding the Importance of Training Data for Self-Driving Cars
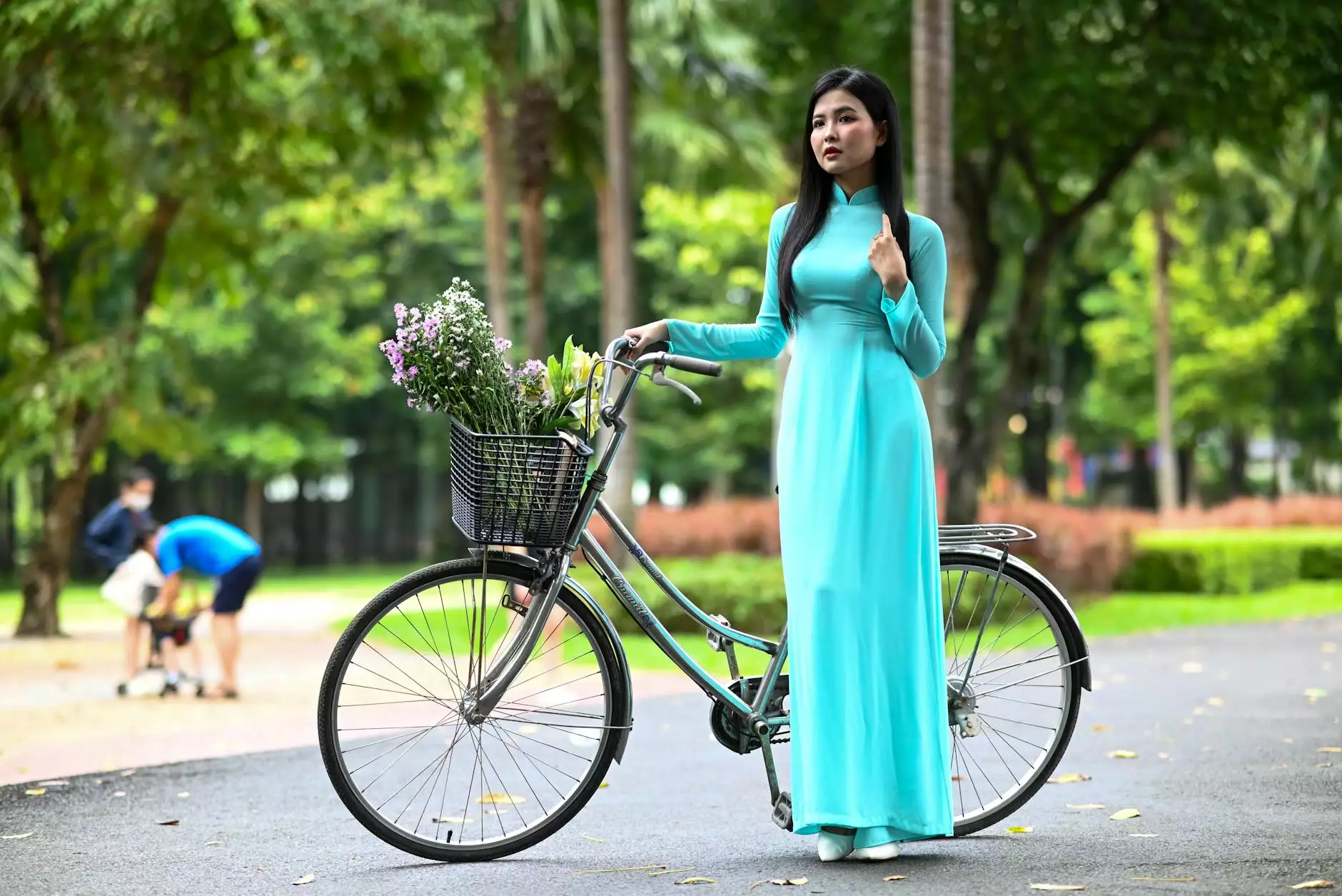
The advent of autonomous vehicles marks a significant evolution in transportation, bringing forth innovations that promise to revolutionize the way we commute. At the core of this technological breakthrough lies an essential element: training data for self-driving cars. In this article, we will delve into the multifaceted nature of training data, its types, significance, challenges in acquisition, and its profound impact on the future of transportation.
The Foundation of Autonomous Driving: What is Training Data?
Training data refers to the extensive datasets used to train machine learning models, allowing them to identify patterns and make informed decisions. In the context of self-driving cars, this data includes a variety of inputs such as:
- Images from cameras used for object detection
- LiDAR point clouds for understanding distances and shapes
- Radar data for detecting objects in various weather conditions
- GPS data for location tracking
- Sensor Fusion combining data from multiple sources for enhanced accuracy
Types of Training Data
To effectively train autonomous vehicles, engineers rely on diverse types of training data:
1. Visual Data
The primary source of information comes from cameras mounted on the vehicles. This visual input helps self-driving systems recognize pedestrians, road signs, lane markings, and other critical elements of the driving environment.
2. Spatial and Temporal Data
LiDAR sensors provide detailed 3D mapping of the surrounding environment. Temporal data helps cars understand how objects move over time, crucial for predicting possible interactions during a drive.
3. Driving Behavior Data
Data regarding human driving behaviors and decisions is vital for algorithm training. Understanding the subtleties of how a human driver reacts to different scenarios assists in developing safer, more intuitive autonomous systems.
The Significance of Training Data
Training data serves as the backbone of machine learning for self-driving cars, influencing several aspects:
1. Safety and Reliability
Training data for self-driving cars plays a critical role in ensuring that these vehicles can safely navigate complex environments. Proper training enables them to avoid accidents, making informed decisions that mimic those of a careful human driver.
2. Vehicle Performance
The quality and diversity of the training datasets directly affect vehicle performance. More diverse datasets lead to better generalization in varying conditions, ultimately enhancing the vehicle's operational capabilities in real-world scenarios.
3. Regulatory Compliance and Public Acceptance
As self-driving technology evolves, it comes under scrutiny from regulatory bodies. Comprehensive training data showcasing safety can help gain public trust and promote smoother regulatory approvals.
Challenges in Acquiring Quality Training Data
While the importance of training data is clear, acquiring high-quality data presents significant challenges:
1. Volume of Data Required
One of the biggest hurdles is the sheer volume of data necessary to train self-driving car models. These vehicles must be exposed to millions of driving scenarios to accurately learn how to react in different environments.
2. Diversity of Conditions
Data must encompass a variety of weather conditions, times of day, and geographical locations. This diversity ensures that the training models can handle unexpected changes in the environment.
3. Edge Cases
Edge cases, or rare driving scenarios, are essential to include in training data. These events, while infrequent, could be critical for safety. Gathering data during such rare occurrences can be incredibly challenging.
Methods of Collecting Training Data
Given the challenges, numerous methods can be employed to gather robust training data:
1. On-Road Data Collection
Using fleets of vehicles equipped with various sensors, companies can capture extensive real-world driving data. These vehicles then collect data over millions of miles driven in multiple conditions.
2. Simulation Environments
Virtual environments allow developers to simulate countless driving scenarios. This method helps create synthetic training data that can fill gaps in real-world data, particularly for edge cases.
3. Crowd-sourced Data
Some companies employ crowd-sourcing to gather data from users. This data can include user feedback and specific driving conditions encountered, enriching the overall dataset.
The Future of Training Data for Self-Driving Cars
As technology progresses, the strategies employed to collect and utilize training data will also evolve:
1. Increased Use of AI in Data Labeling
Automating the data labeling process using advanced AI can save time and resources. As AI gets better at understanding context, it can help categorize vast datasets more accurately.
2. Collaborative Data Sharing
Partnerships among automotive companies and tech firms may lead to collaborative platforms for data sharing. Such cooperation could significantly enhance the breadth and quality of training datasets.
3. Focus on Ethical Data Collection
As the importance of data privacy grows, it will be crucial for organizations to gather training data ethically, ensuring compliance with regulations and maintaining public trust.
Conclusion: The Role of Training Data in Shaping Autonomous Driving
In conclusion, training data for self-driving cars is not just a peripheral component; it is the lifeblood of the autonomous vehicle revolution. The future of transportation hinges on the quality, diversity, and ethical collection of this data. As industry players continue to innovate and address the challenges ahead, the potential benefits of self-driving technology will soon become a reality, transforming our roads and societal mobility.
To stay ahead in this rapidly evolving industry, organizations like Keymakr in the fields of Home Services and Keys & Locksmiths can draw valuable lessons from the meticulous collection and application of data in developing effective and safe services, ensuring that they remain relevant and competitive in the face of advancing technologies.
training data for self driving cars