The Essential Guide to Image Labeling: Boosting Your Business with Effective Data Annotation
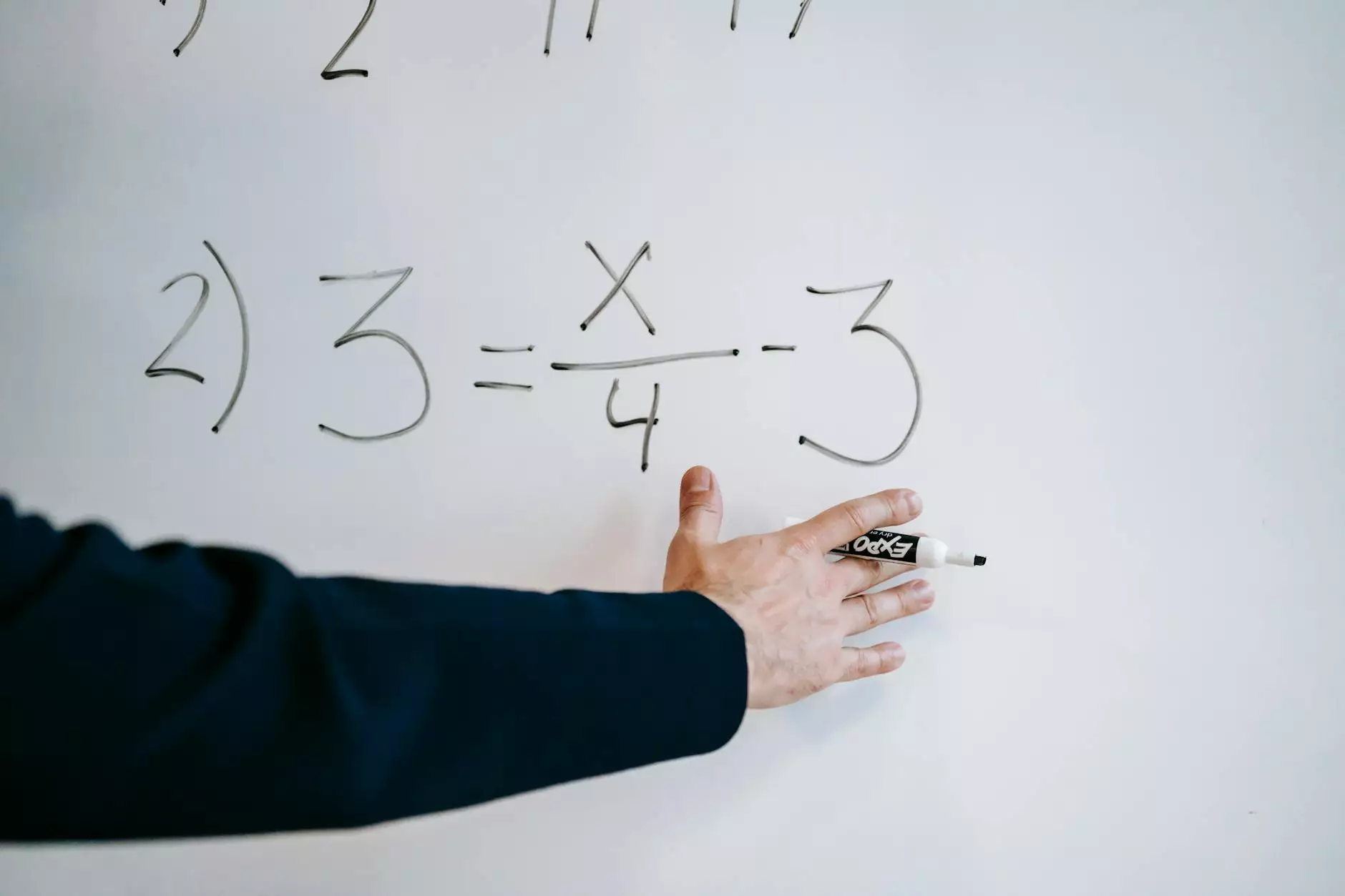
In today's data-driven landscape, businesses are increasingly leveraging machine learning and artificial intelligence to streamline operations and enhance customer experiences. A crucial part of this process is image labeling, a technique vital for training machine learning models. This article delves into the intricacies of image labeling, explaining its significance, benefits, and best practices, while showcasing how platforms like KeyLabs.ai can aid organizations in mastering this art.
Understanding Image Labeling
Image labeling refers to the process of identifying and tagging specific objects within an image. This task is essential in various domains such as autonomous driving, healthcare diagnostics, and retail inventory management. The labeled data serves as the foundation for training algorithms, enabling them to recognize patterns and make predictions based on new, unlabeled data.
The Technical Aspect of Image Labeling
- Data Collection: Gather a diverse set of images that accurately represent the problem space.
- Annotation Tools: Utilize advanced data annotation tools to facilitate the efficient labeling of images.
- Quality Control: Implement quality control measures to ensure high accuracy in the labeling process.
- Model Training: Utilize the labeled images to train machine learning models.
The Importance of Image Labeling for Businesses
Businesses that incorporate image labeling into their processes stand to gain numerous advantages:
1. Enhanced Model Accuracy
The accuracy of machine learning models heavily relies on the quality of the training data. By ensuring that images are precisely labeled, businesses can significantly improve the performance of their algorithms. This leads to more reliable predictions and better decision-making.
2. Increased Efficiency
With automated image labeling tools, businesses can expedite the data preparation phase. This results in faster model training times and less downtime, ultimately leading to quicker deployments of AI solutions.
3. Competitive Advantage
Companies that effectively employ data annotation techniques can differentiate themselves from competitors. By utilizing high-quality annotated data, businesses can develop unique solutions tailored to their customers' needs, enhancing overall satisfaction and loyalty.
4. Diverse Applications
The applications of image labeling are vast. From healthcare—where labeled images assist in diagnostic models—to retail, where they inform inventory and customer behavior analysis, the potential uses are limited only by the imagination.
Choosing the Right Data Annotation Platform
When considering a data annotation platform for image labeling, it is critical to select one that meets your specific needs. Here are key features to look for:
- User-Friendly Interface: A platform should offer an intuitive UI that makes the labeling process straightforward.
- Scalability: It should easily handle varying data volumes as your business grows.
- Collaboration Tools: The ability to collaborate with teams across different locations enhances productivity.
- Automation Features: Look for tools that provide automated labeling options to save time and reduce human error.
- Robust Support: Quality customer support can ease the transition and assist in resolving any technical issues.
Best Practices for Image Labeling
To maximize the benefits of image labeling, it’s imperative to follow best practices in the annotation process. Here’s how you can do it:
1. Define Clear Labeling Guidelines
Your team should have a comprehensive understanding of what each label entails. Clear guidelines reduce inconsistencies and ensure all annotators are on the same page.
2. Use High-Quality Images
High-resolution images help in achieving accurate annotations. Blurry or low-quality images can lead to mislabeling, adversely affecting model training.
3. Employ Quality Assurance Techniques
Regularly review the labeled data for accuracy. Implementing peer reviews or automated checks can catch errors early.
4. Continuous Learning and Adaptation
The field of image labeling is continuously evolving. Stay updated with the latest techniques and tools to maintain efficiency and accuracy.
The Future of Image Labeling
As technology advances, so does the field of image labeling. Innovations such as artificial intelligence and machine learning are paving the way for smarter annotation tools that can reduce manual effort significantly. Here are some trends to watch:
1. Automation of Labeling Processes
With the emergence of natural language processing (NLP) and computer vision technologies, automated image labeling is becoming more accurate and efficient. Businesses can look forward to leveraging these technologies to minimize human intervention in the labeling process.
2. Integration with Other AI Technologies
Image labeling platforms are increasingly being integrated with various AI technologies to provide a seamless workflow. This integration can enhance the overall data analysis process and lead to richer insights.
3. Enhanced Collaboration Tools
Future platforms will likely incorporate advanced collaborative features, allowing teams to work together more effectively, regardless of their geographic locations.
Conclusion
In summary, image labeling is not just a technical requirement; it is a strategic imperative for businesses looking to harness the power of machine learning and artificial intelligence. Adopting effective data annotation practices can lead to significant improvements in model accuracy, operational efficiency, and customer satisfaction.
Partnering with a robust platform like KeyLabs.ai ensures you have the tools needed to excel in this domain. Embrace the future of data annotation, and watch your business thrive in an increasingly competitive marketplace.