Mastering Labeling Training Data for Enhanced AI Performance
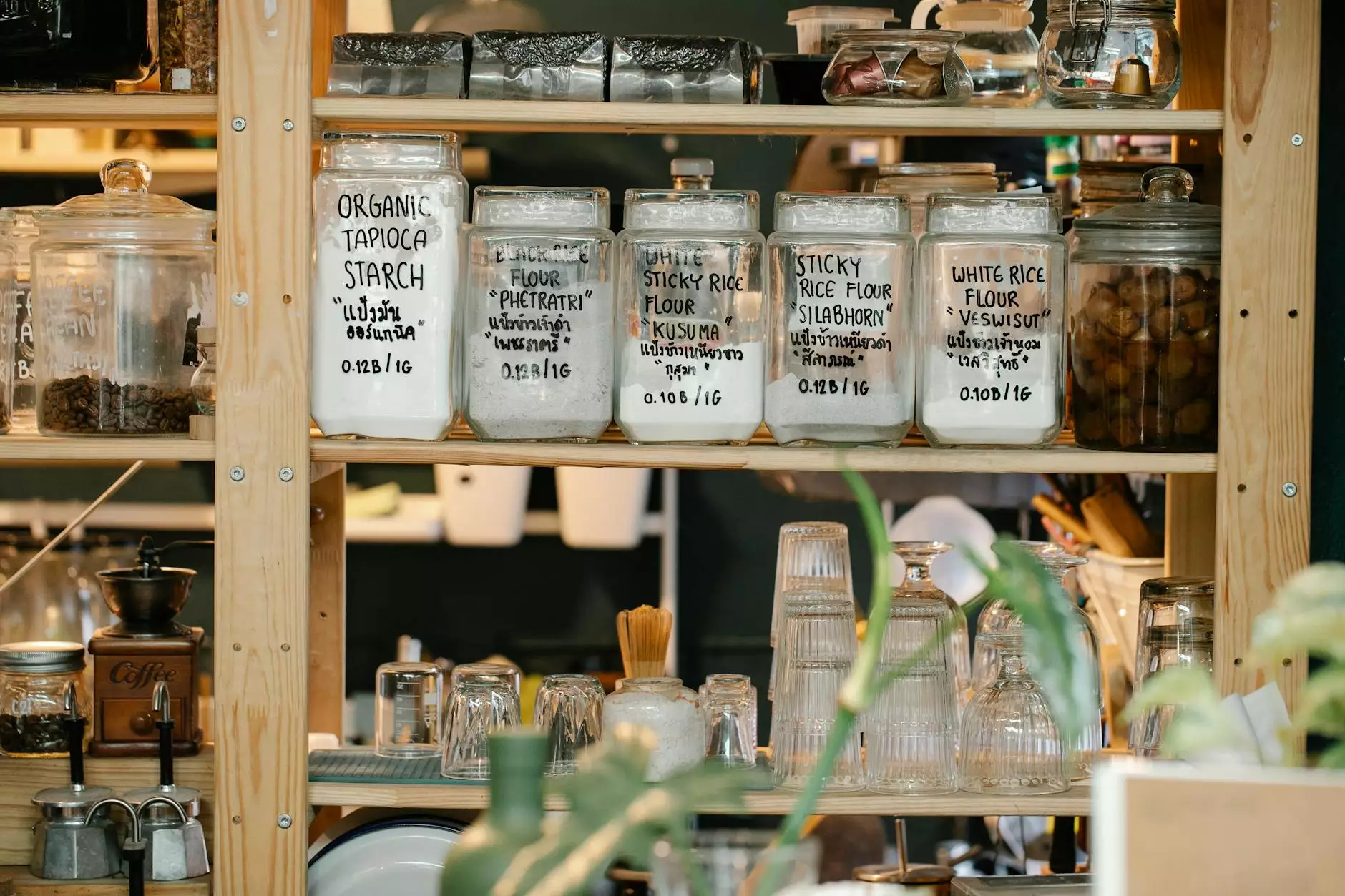
In the rapidly evolving world of artificial intelligence (AI), the importance of labeling training data cannot be overstated. It serves as the backbone of machine learning algorithms, enabling them to learn from vast datasets and make accurate predictions. In this comprehensive article, we will delve into the nuances of labeling training data, the advantages of utilizing advanced data annotation tools and platforms, and how Keylabs.ai can be your ultimate partner in achieving impeccable AI outcomes.
Understanding the Importance of Labeling Training Data
At its core, labeling training data involves assigning meaningful and informative tags to raw data. This process is crucial for teaching machine learning models how to interpret information correctly. Without properly labeled data, models can become confused, yielding inaccurate results and diminishing their utility. Here are some key reasons why labeling is essential:
- Enhances Model Accuracy: High-quality labeled data allows models to learn nuanced patterns, increasing their predictive accuracy.
- Facilitates Model Training: Labeled datasets provide a clear structure for machine learning algorithms to understand input-output relationships.
- Improves Generalization: Well-labeled data helps models generalize better to unseen data, making them more effective in real-world applications.
- Accelerates Development: Clear, concise labels expedite the training process, reducing the time developers spend fine-tuning algorithms.
Types of Labeling in Data Annotation
The process of labeling training data can take various forms, depending on the type of data being processed. Below are the primary types of labeling utilized in data annotation:
1. Image Annotation
Image annotation is prevalent in computer vision applications. It involves tagging images with relevant labels, such as identifying objects, segmenting regions, or classifying actions. Common methods include:
- Bounding Box: Drawing boxes around objects in images for detection tasks.
- Semantic Segmentation: Labeling each pixel in an image to distinguish between different regions.
- Instance Segmentation: Identifying individual objects within images at the pixel level.
2. Text Annotation
Text annotation involves labeling textual data through various methods, tailored for natural language processing (NLP) tasks. This may include:
- Sentiment Analysis: Identifying the sentiment expressed in text (positive, negative, neutral).
- Named Entity Recognition: Extracting specific entities like names, dates, and locations.
- Text Classification: Assigning categories to documents based on content.
3. Audio and Video Annotation
Audio and video files require specialized labeling to facilitate recognition and processing, such as:
- Speech Recognition: Transcribing spoken words into text for voice-activated applications.
- Activity Recognition: Identifying activities from video frames, useful for surveillance and monitoring.
Challenges in Labeling Training Data
While labeling training data is crucial, the process comes with its own set of challenges. Recognizing these challenges can help organizations mitigate risks and streamline their data annotation processes:
Quality Assurance
Ensuring consistent quality in data labeling is essential. Inconsistent labels can lead to confusion and lesser model performance. Implementing strict quality control measures helps mitigate this issue.
Scalability
The demand for labeled data is growing exponentially. As businesses scale, so does the need for more extensive datasets. Efficient workflows and automation are critical for keeping up with this demand.
Data Privacy and Compliance
Labeling sensitive data like personal information poses challenges regarding privacy laws and compliance. It is crucial to establish rigorous data handling protocols to protect user information.
Leveraging Technology for Efficient Labeling
Embracing technology is fundamental to overcoming these challenges in labeling training data. Advanced data annotation tools and platforms can significantly improve the accuracy and efficiency of data labeling tasks. Here’s how:
Automated Annotation Tools
Using AI-driven automated annotation tools can streamline the labeling process. These tools leverage pre-trained models to suggest labels, reducing manual effort and expediting the overall process.
Collaborative Annotation Platforms
Collaborative platforms enable teams to work together on data annotation projects, ensuring that different perspectives contribute to the accuracy of labels while enhancing productivity.
Quality Control Mechanisms
Advanced platforms come equipped with built-in quality control mechanisms, like review workflows and validation checks, ensuring high-quality labels through robust oversight.
Keylabs.ai: Your Partner in Data Annotation
When it comes to data annotation, Keylabs.ai stands out as an industry leader. By providing state-of-the-art data annotation tools and platforms, Keylabs.ai ensures that your AI projects are built on a foundation of high-quality labeling training data. Here’s what makes Keylabs.ai exceptional:
1. Comprehensive Annotation Solutions
Keylabs.ai offers a wide range of data annotation solutions tailored to various data types. From image and text to audio and video, their platform supports diverse applications.
2. High-Quality Data Assurance
With rigorous quality control protocols in place, Keylabs.ai guarantees the accuracy and reliability of their annotated datasets, ensuring your models perform optimally.
3. Scalability and Flexibility
The scalable nature of Keylabs.ai’s platform allows businesses to adapt to growing data needs. Whether you require small batches or large-scale annotations, their infrastructure is designed to meet your requirements.
4. User-Friendly Interface
Keylabs.ai prioritizes user experience with an intuitive interface that simplifies the annotation process, allowing teams to focus on their core tasks without cumbersome navigation.
5. A Commitment to Data Security
Recognizing the significance of data privacy, Keylabs.ai adheres to stringent data protection policies, ensuring compliance with all relevant regulations while handling your data responsibly.
Best Practices for Labeling Training Data
To achieve the best results in labeling training data, consider adopting the following best practices:
- Define Clear Guidelines: Establish precise labeling instructions to ensure consistency across your datasets.
- Invest in Training: Provide proper training for annotators to reduce errors and foster a deep understanding of the labeling criteria.
- Implement a Review Process: Create a systematic review process to catch errors and maintain high data quality.
- Utilize Feedback Loops: Encourage feedback from annotators to refine guidelines and improve workflow efficiency over time.
The Future of Labeling Training Data
As AI technologies continue to advance, the landscape of labeling training data is also expected to evolve. Emerging trends include:
1. Increased Use of Machine Learning
Enhanced machine learning algorithms will increasingly contribute to automated labeling, reducing the need for manual input and enabling faster data processing.
2. Ethical Considerations in Data Annotation
As the demand for annotated data grows, ethical considerations around data labeling practices, including fairness and bias, will become increasingly important in shaping industry standards.
3. Integration with AI Workflows
Seamless integration with AI development workflows will become paramount, allowing organizations to unify their data annotation and model training processes for enhanced efficiency.
Conclusion
The significance of labeling training data in the realm of artificial intelligence and machine learning is undeniable. With the right tools and strategies, businesses can dramatically improve their AI capabilities. Partnering with Keylabs.ai gives you the competitive edge needed to thrive in this data-driven era. Invest in high-quality data annotation today to ensure the success of your AI projects tomorrow.